With the widespread use of deep learning models in practical applications, there are increasing concerns that these models could perpetuate or amplify societal biases and discrimination if not properly regulated. In this talk, I will discuss approaches to enhance the fairness of deep learning models from both data and model perspectives, highlighting their applications in computer vision and natural language processing tasks. Our framework is theoretically grounded, effective in balancing model performance and fairness, and computationally efficient.
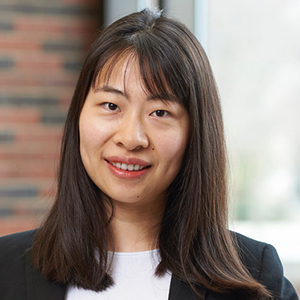
Xiaoqian Wang is an assistant professor of electrical and computer engineering at Purdue University. She received her Ph.D. degree from the University of Pittsburgh in 2019 and her B.S. degree from Zhejiang University in 2013. Her research focuses on designing novel machine learning models for interpretability, fairness, and robustness. She also works at the intersection of machine learning, bioinformatics, and healthcare. She is the recipient of an NSF CAREER award in 2022, an AAAI Distinguished Paper Award in 2023, and is an IEEE senior member.