There are over 9.9 million new cases of dementia each year worldwide, implying one new case every 3.2 seconds. The increased aging population will further exaggerate this number. The learning tasks in modeling neurodegenerative diseases are facing unique challenges from both data sparseness and complicated non-linear interactions between feature variables and target responses.
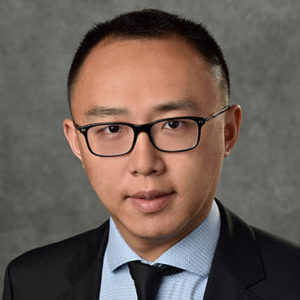
In this talk, Dr. Jiayu Zhou will present his recent research on artificial intelligence approaches for decoding the mechanism of aging and neurodegenerative diseases using insights jointly derived from data and knowledge.
For learning tasks from imaging markers, his group developed the subspace network, which is an efficient deep modeling approach for non-linear multi-task learning from small datasets. Each layer of the subspace network performs multi-task learning to improve upon the predictions from the last layer via sketching a low-dimensional subspace to perform knowledge transfer among learning tasks. Empirical results demonstrate that the subspace network quickly picks up the correct parameter subspaces, and outperforms state-of-the-arts in predicting neurodegenerative clinical scores using the information in brain imaging.
For learning with language markers, his group introduced a novel reinforcement learning framework to train a dialogue agent that conducts efficient conversations with elder subjects and identifies early dementia. The agent is trained to sketch disease-specific lexical probability distribution, and thus to converse in a way that maximizes the diagnosis accuracy and minimizes the number of conversation turns. The results show that while using only a few turns of conversation, their framework can significantly outperform state-of-the-art supervised learning approaches.
Dr. Jiayu Zhou is currently an associate professor in the Department of Computer Science and Engineering at Michigan State University. He received his Ph.D. in computer science from Arizona State University in 2014. He has a broad research interest in large-scale machine learning, data mining, and biomedical informatics, with a focus on transfer and multi-task learning.
Dr. Jiayu Zhou’s research has been funded by the National Science Foundation, National Institutes of Health, and Office of Naval Research. He has published more than 100 peer-reviewed journal and conference papers in data mining and machine learning.
Dr. Zhou is a recipient of the National Science Foundation CAREER Award (2018). His papers received the Best Student Paper Award in the 2014 IEEE International Conference on Data Mining (ICDM), the Best Student Paper Award at the 2016 International Symposium on Biomedical Imaging (ISBI), and Best Paper Award in the 2016 IEEE International Conference on Big Data (BigData).