The next generation of supercomputers will be exascale (1018 floating-point operations per second) computer systems. These systems will help scientists and engineers tackle extremely complex high-performance computing (HPC) and artificial intelligence (AI) problems for critical societal challenges, such as climate change, water management, advanced manufacturing, and vaccine and drug design. However, due to the gap between ever-increasing compute power and limited storage capacity and I/O bandwidth, scientists and engineers must create intelligent and effective methods to efficiently manage massive amounts of data generated by HPC and AI applications for fast storage and transmission.
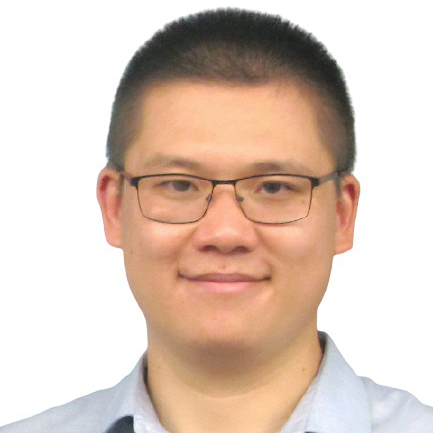
This talk will introduce our promising solution — error-bounded lossy compression — that can significantly reduce the data sizes while maintaining high data fidelity for post-analyses in HPC and AI applications. The talk will cover the design, optimization, and use of our error-bounded lossy compression to advance HPC and AI systems (e.g., GPU-based heterogenous systems) for large-scale data processing applications (e.g., HPC simulations and AI model training).
Dr. Dingwen Tao is an assistant professor in School of EECS at Washington State University. He received his B.S. degree in Mathematics from University of Science and Technology of China in 2013 and his Ph.D. degree in Computer Science from University of California, Riverside in 2018. Prior to joining WSU, he worked at the University of Alabama and multiple Department of Energy national laboratories. His research interests include high-performance computing (HPC), parallel and distributed systems, and large-scale machine learning. He has published more than 50 papers in many top-tier conferences and journals, including SC, ICS, HPDC, VLDB, ICDE, PPoPP, IPDPS, PACT, Cluster, DAC, BigData, ICPP, TPDS, TC, JPDC, etc. He is the receipt of 2021 R&D100 Award, 2020 IEEE-CS TCHPC Early Career Researchers Award for Excellence in HPC, 2020 NSF CRII Award, and 2017 UCR Dissertation Year Program Award. His research has been supported by NSF, DOE, NOAA, Xilinx, and AMD.