A gap has emerged in many domains between the performance of the most predictive models, which are typically deep neural networks, and models whose parameters are readily interpretable. This gap raises questions concerning which assumptions embedded in deep learning models / training algorithms allow them to learn models that generalize, what such assumptions correspond to semantically in particular domains, and how we might use such implicit semantics to gain new knowledge about a domain.
Jonathan Warrell of Yale University will discuss these issues from a PAC-Bayes viewpoint, particularly focusing on how model architectures, incorporation of prior knowledge, and compressibility/complexity control can be motivated by these considerations in the context of genomics and neuroscience.
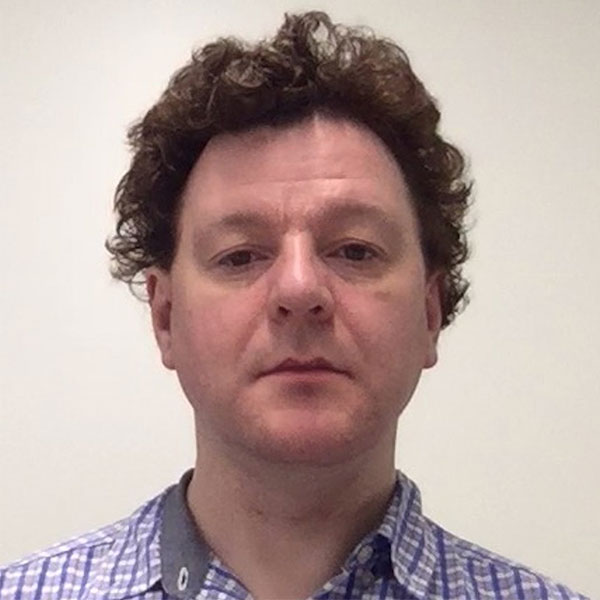
He will outline how such considerations have led to specific model architectures and analytic methods he has developed in confronting problems in a range of domains. These include developing integrated models of genetic risk for psychiatric disorders and cognition as part of the NIH’s PsychENCODE consortium (including genetic, epigenetic, cellular, and brain imaging data), detecting positive and negative selection in cancer, and identifying latent evolutionary processes in genomics and cultural domains.
Warrell will also discuss how techniques from PAC-Bayes analysis, probabilistic programming and dependent type theory can be used to provide a theoretical basis for the models he introduces, and derive higher-order generalization bounds, which can in turn motivate novel training algorithms.
Jonathan Warrell is a postdoctoral associate research scientist in the Computational Biology and Bioinformatics program at Yale University, working with Mark Gerstein. He has published extensively in computational biology, machine learning, computer vision, and theoretical biology and evolution. He is currently a member of several large-scale genomics consortia, including ENCODE, PsychENCODE, and PCAWG (Pan-Cancer Analysis of Whole Genomes), and his work has been featured in the journals Science and Cell, as well as conferences such as CVPR, ECCV, and ISMB.
Jonathan has held postdoctoral positions in computer vision and machine learning at University College London and Oxford / Oxford Brookes Universities, and computational biology and genomics at the University of Cape Town and Yale University. He began his academic career in music theory and holds a BA in music from Cambridge, an MA, and Ph.D. from King’s College London in music theory and analysis, and an MSc in computer science from University College London. His current research areas include integrated models of genetic risk in psychiatric genomics, neuroscience and cancer, interpretable machine learning, statistical learning theory, and generalized evolutionary models of gene networks, cancer, and cultural processes.
Contact Ginny Watterson for Zoom link.