High noise and intermediate scale are two well-known major hurdles in Noisy Intermediate-Scale Quantum (NISQ) computing. The recent breakthroughs have shed light on addressing the scalability issue — evidenced by IBM with the unveiled 433-qubit Osprey processor last year, which makes quantum computing promising for a wide range of applications. However, the inherent quantum noise brings great challenges in enabling quantum computing to be deployable and used for practical applications.
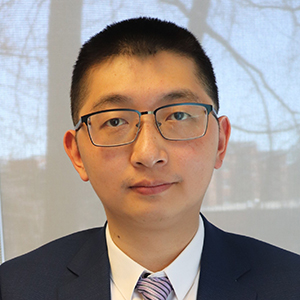
This talk will take quantum learning as a vehicle to demonstrate the opportunities and challenges of practical quantum applications in the NISQ era. I will first talk about the QuantumFlow design to exploit the potential quantum advantage for quantum learning tasks. Then, I will introduce the challenges brought by quantum noise, which derives us from developing quantum neural network compression. Finally, I will talk about the challenges brought by one important, but not well-studied property of NISQ devices, that is, the quantum noise is not stable. Overall, we aim to develop a stack to provide practical quantum learning on NISQ devices.
Dr. Weiwen Jiang is an Assistant Professor in the ECE department at George Mason University. He was a Postdoctoral Associate at the University of Notre Dame. He received the Ph.D. degree from Chongqing University in 2019. From 2017 to 2019, he was a research scholar at the University of Pittsburgh. His research works have won Best Paper Awards in IEEE TCAD’21, ICCD’17, and NVMSA’15. He is the receipt of four Best Paper Nominations in ASP-DAC’16, DAC’19, CODES+ISSS’19, ASP-DAC’20, and the Top Winning Awards at IEEE Services Hackathon. He is serving as associate editor at IEEE Transactions on Circuits and Systems II and program track chair at IEEE Quantum Week 2023.